Due to the high quality of the IFI CLAIMS patent data, especially the translations, we were able to extract the most relevant features for the algorithm very easily. The excellent coverage from IFI gave us the opportunity to train our algorithm on millions of data points from various technology fields and jurisdictions. We focused on identifying “weak” patents with a very low probability of being granted. And the results speak for themselves:
Figure 1
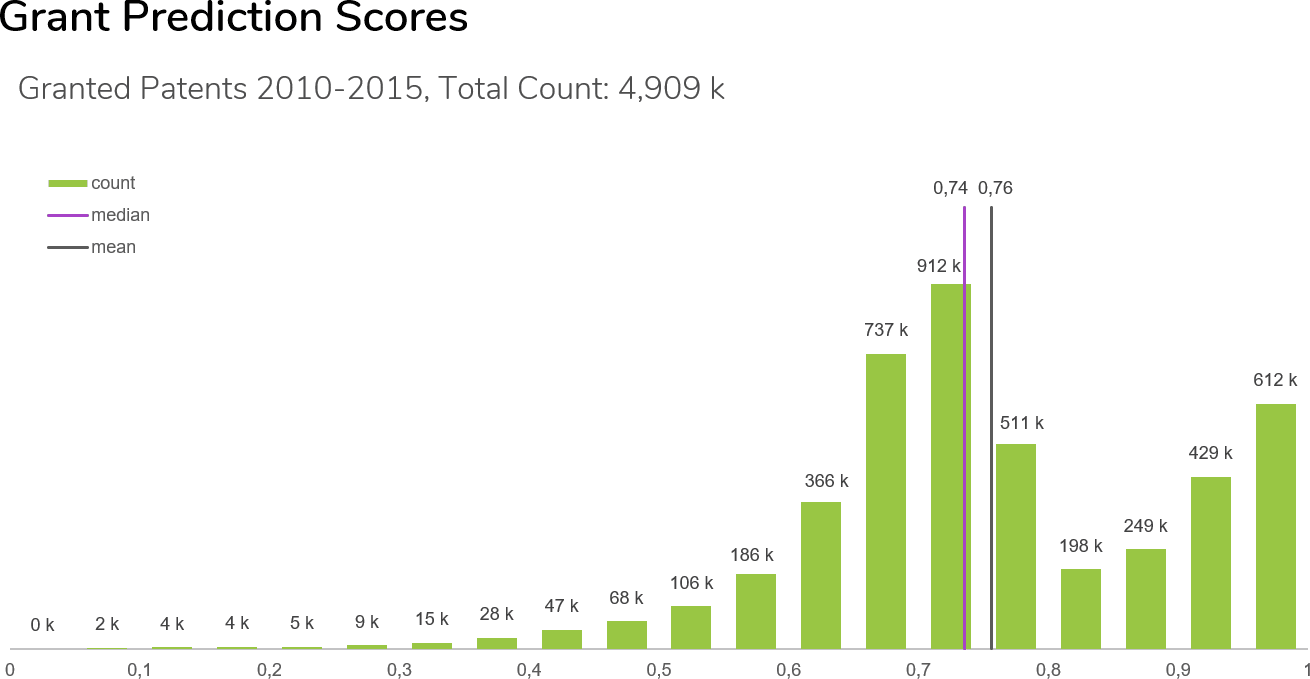
What we see in Fig 1 is a data sample of applications from 2010 to 2015 that have been granted. The granting probability distribution for those patents is skewed to the right towards higher probabilities ex ante.
Most importantly, very few patents that were eventually granted received a low grant probability score of 30 percent or lower in the predictive analysis. This indicates a very low error rate in identifying patents that won’t be granted. Fig 2 shows the error rate with different levels of probability used to determine the cut-off point.
Figure 2
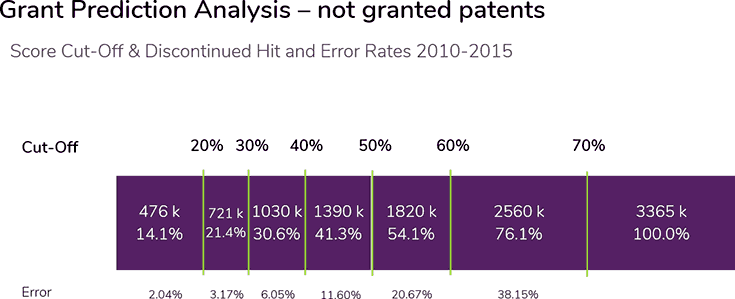
The graph above uses a 30 percent granting probability or lower as the cut-off point to decide whether to discontinue a patent filing. Using this analysis, applicants would have identified 721,000 patents or 21.4 percent of all non-granted patents at the date of publication—with an error rate of just 3.17 percent.
We have calculated these error rates for different industries and individual companies and obtained very consistent outcomes, with correct prediction rates between 97 and 99 percent for a large chunk of all patents that did not end up being granted. With this low number of errors, IP departments can use our probability scores in the process of cleaning up patent portfolios and when reevaluating recent applications.
Our algorithm also adds significant value in M&A situations for younger companies. In these cases, most of the patent portfolio are still in the application stage. Our algorithm can help investors who do not have a deep knowledge of the underlying inventions to assess the value of the patent portfolio of the target company. After all, a patent application that is not granted is not worth much.